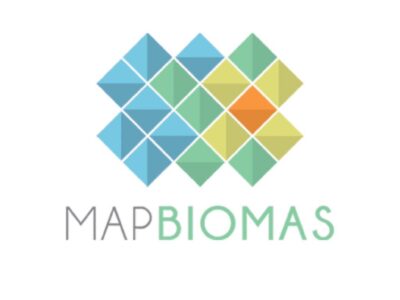
Santos et al – Assessing the Wall-to-Wall Spatial and Qualitative Dynamics of the Brazilian Pasturelands 2010–2018, Based on the Analysis of the Landsat Data Archive
In this study, the spatial-temporal dynamics of pasture quality in Brazil between 2010 and 2018 were mapped and evaluated, considering three degradation classes: Absent (D0), Intermediate (D1), and Severe (D2. There was no variation in the total area occupied by pastures in the evaluated period, despite the accentuated spatial dynamics.
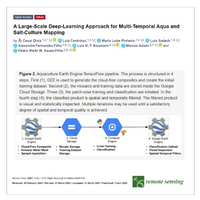
Cesar et al. – A Large-Scale Deep-Learning Approach for Multi-Temporal Aqua and Salt-Culture Mapping
Aquaculture and saliculture are relevant economic activities in the Brazilian Coastal Zone (BCZ). However, automatic discrimination of such activities from other water-related coverages/uses is not an easy task. In this sense, convolutional neural networks (CNN) have the advantage of predicting the class label of a given pixel, providing as input a local region (patches or named chips) around that pixel. Both the convolutional nature and the semantic segmentation capability provide the U-Net classifier with the ability to access the "context domain" instead of just isolated pixel values. Supported in the context domain, we present the results of the analyzes.
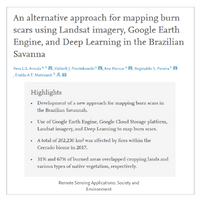
Arruda et al – An alternative approach for mapping burn scars using Landsat imagery, Google Earth Engine, and Deep Learning in the Brazilian Savanna
In this study, we developed an alternative approach for mapping burned areas in the Cerrado biome in Brazil, using Landsat imagery and Deep Learning algorithm, implemented on the Google Earth Engine and on the Google Cloud Storage platform.
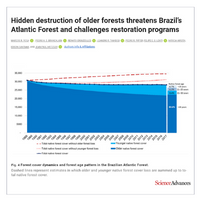
Rosa et al. – Hidden destruction of older forests threatens Brazil’s Atlantic Forest and challenges restoration programs.
Understanding the dynamics of native forest loss and gain is critical for biodiversity conservation and ecosystem services, especially in regions experiencing intense forest transformations. We quantified native forest cover dynamics on an annual basis from 1990 to 2017 in Brazil's Atlantic Forest.
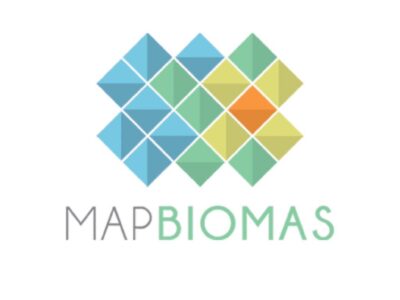
Souza et al. – Reconstructing Three Decades of Land Use and Land Cover Changes in Brazilian Biomes with Landsat Archive and Earth Engine.
In this study, we described a novel approach and the results achieved by a multi-disciplinary network called MapBiomas to reconstruct annual land use and land cover information between 1985 and 2017 for Brazil, based on random forest applied to the Landsat archive using Google Earth Engine.
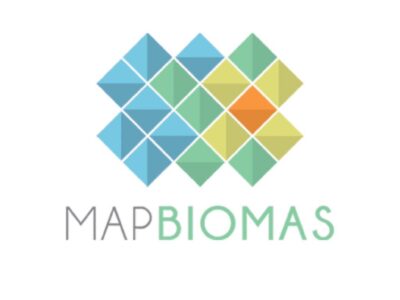
Fendrich et al. – Disclosing contrasting scenarios for future land cover in Brazil: Results from a high-resolution spatiotemporal model
In this work, we constructed a model to evaluate the possible consequences of policy actions on land cover dynamics in the near future at a high-resolution scale.
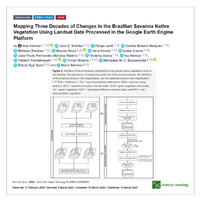
Alencar et al – Mapping Three Decades of Changes in the Brazilian Savanna Native Vegetation Using Landsat Data Processed in the Google Earth Engine Platform.
The Brazilian Cerrado represents the largest savanna in South America, and the most threatened biome in Brazil, owing to agricultural expansion. To assess the native Cerrado vegetation (NV) areas most susceptible to natural and anthropogenic change over time, we classified 33 years (1985-2017) of Landsat imagery available in the Google Earth Engine (GEE) platform.
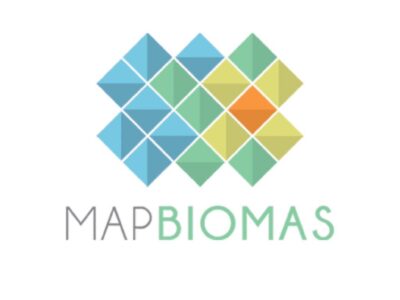
Nunes et al – Unmasking secondary vegetation dynamics in the Brazilian Amazon
This article uses MapBiomas annual land cover time series data to generate the first estimates of the extent of VS, age, and net carbon absorption in the Brazilian Amazon between 1985 and 2017.
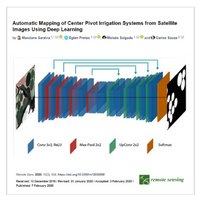
Saraiva et al- Automatic Mapping of Center Pivot Irrigation Systems from Satellite Images Using Deep Learning
In this paper, we propose a method to automatically detect and map center pivot irrigation systems using U-Net, and image segmentation convolutional neural network architecture applied to a constellation of PlanetScope images from the Cerrado biome of Brazil. Our objective is to provide a fast and accurate alternative to map center pivot irrigation systems with very high spatial and temporal resolution imagery.
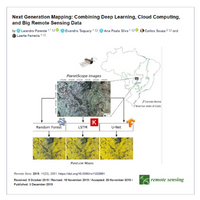
Parente et al – Next Generation Mapping: Combining Deep Learning, Cloud Computing, and Big Remote Sensing Data.
This study evaluated, based on thousands of PlanetScope images obtained over a 12-month period, the performance of three machine learning approaches (random forest, long short-term memory - LSTM, and U-Net). We applied these approaches to mapped pasturelands in a Central region in Brazil.